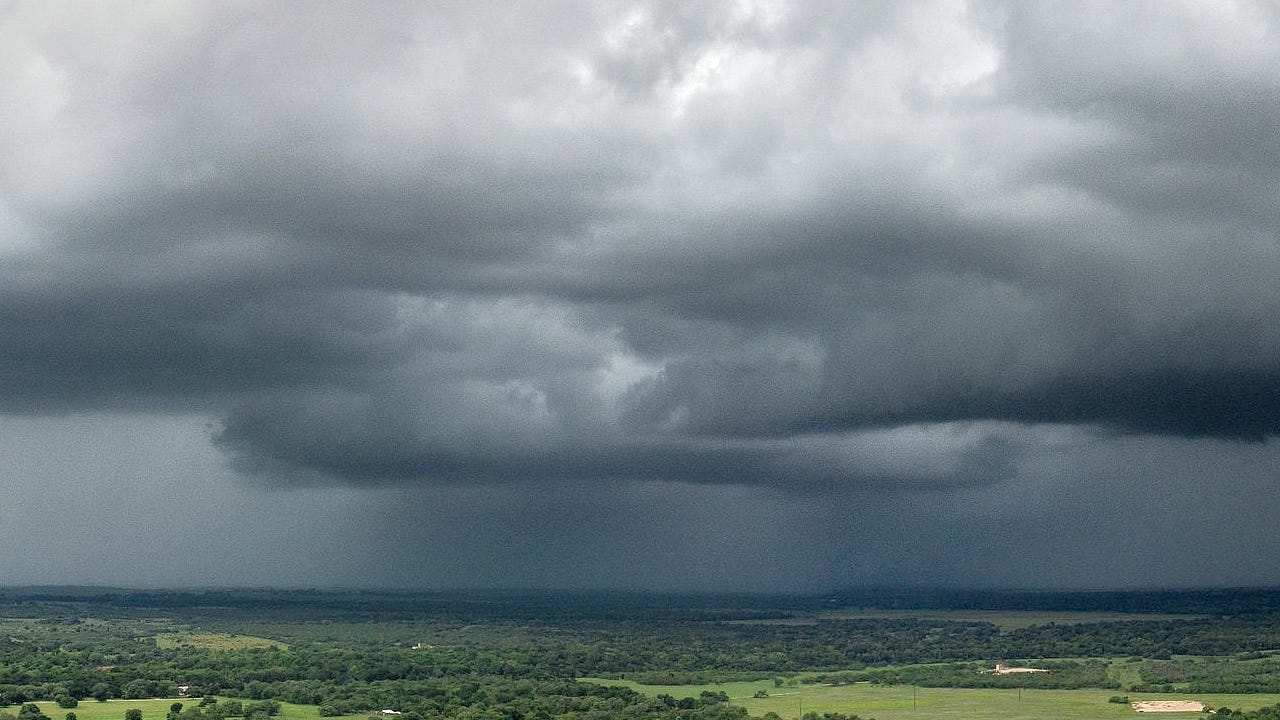
Forecasting different time frames requires different approaches and different skills. Some things we can more or less see coming and can be predicted with confidence. Other things are predictable primarily in the sense that they conform to historical patterns. Others are really just informed speculation.
It’s easy to see some things coming. You don’t need special insight to know that dark clouds probably mean a storm is on its way. Good forecasters can see the signs of an approaching storm before they’re obvious to everyone else. Because slight variations in conditions today can make a substantial difference in the weather tomorrow—the famous “butterfly effect”—it may be practically impossible to see forecast the weather precisely more than a couple of weeks in advance.1 But while you can’t see specific weather systems coming a year ahead of time, you can still assume the weather will be similar to the weather in previous years at the same time of year. The farther ahead you try to forecast, the more speculative and less certain your forecasts become. As the climate changes, regular weather patterns will break down. Eventually, the future becomes too obscure to see at all.
Last year, Our World in Data tasked forecasters at Good Judgment and Metaculus—I was part of the Metaculus group—to forecast 20 key indicators of global development across the next 100 years. We were asked to forecast the value of each indicator 1, 3, 10, 30, and 100 years in the future (Metaculus’ summary of our forecasts is here). One striking thing about the project was how different the task of forecasting the same metric across different time frames was. One of the indicators we were asked to forecast, for example, was global population. Global population can be predicted with a high degree of confidence in the short term because it generally changes in a smooth, gradual way. Year-to-year variations are such a small percentage of the total that they’re barely visible on a global population graph. Wars and famines barely dent the general trend. The COVID pandemic makes the global population harder to predict in the short term—but only marginally—than it would normally be.
But global population growth isn’t actually linear; it’s slowing because fertility rates are coming down faster than life expectancy is increasing. Eventually, if global fertility falls below the replacement rate of about 2.1 live births per woman, the population will peak and begin to decline. Cultural change and medical innovation will inevitably affect those trends in ways that we can only partially anticipate. Over time a small difference in the rate at which global population growth slows could cause a large deviation from the apparent population trend. The chance of a catastrophe or technological breakthrough that affects the global population may be small over the next few years, but it will start to become significant over the next 30 years.
When we look ahead 100 years, we enter the realm of science fiction. One hundred years ago was before antibiotics, nuclear weapons, the discovery of DNA, space travel, computers, and the internet. It was before WWII, the Holocaust, the Chinese Revolution, decolonization, and the collapse of the Soviet Union. A lot of history will happen in the next 100 years. We’re likely to develop AI that transforms society in unpredictable ways. Life expectancy could rise dramatically as we learn to slow or even prevent aging. Ectogenesis technology could allow embryos to gestate artificially outside the human body. New technology could fundamentally alter what it means to be human. A global catastrophe could have left humanity on the brink of extinction. Metaculus’ professional forecasters thought the global population in 2122 could plausibly range from close to zero to 20 billion—my own guess is that population will peak in the middle of this century before falling to about 6 billion by 2122—but our best guesses about the population 100 years from now are really guesses.

Forecasting different time frames, in other words, requires different approaches and different skills. We can think about the future as falling into three conceptually distinct zones, according to how predictable the subject we’re forecasting is and how far ahead we’re looking:
imminent futures (which are primarily determined by events already happening)
predictable futures (which roughly follow recent historical patterns)
speculative futures (which could accord with one of a wide range of scenarios)
The line between these zones isn’t sharp, because different elements of a complex question may be governed by different dynamics. Looking into the future is a bit like trying to see through a haze. Where visibility is good, we can see objects clearly fairly far in advance; where it’s poor we will struggle to make sense of dim shapes.
Imminent futures are like the weather in the sense that we can see them coming, even when we don’t understand why they’re happening. Some future events are literally scheduled to happen—and even marked on calendars—although anyone who has ever made a plan knows it won’t necessarily happen with 100% certainty. The weather itself may be imminent in this sense for maybe just two weeks, but more stable, regular systems can be imminent much farther in advance. The Our World in Data metrics were imminent 1, 3, or even 10 years ahead of time, in the sense that the things that would determine them had already happened or were already happening. Other systems—like the orbits of the planets—are even more stable and can be confidently predicted much farther in advance. Forecasting imminent futures primarily consists of extrapolating current trends and can be done with relatively high certainty.

When futures are no longer imminent in the sense that they follow from current conditions, they may be predictable in the same way the climate is predictable. We can’t see a storm coming a year from now, but we can still say how often storms occur in a particular place at a particular time. Forecasting predictable (but not imminent) futures is largely a matter of applying patterns from comparable pasts to future situations. It depends upon the ability to distinguish persistent historical patterns from random noise. The metrics that were the subject of the Our World in Data questions were broadly predictable in this sense 10 to 30 years ahead of time. Predictable futures are less certain than imminent futures, but skilled forecasters should still be able anticipate them with some confidence.
Over longer time frames, even long-standing historical patterns begin to break down. Small deviations from the current apparent trajectory add up. Rare, transformative shocks become likely. We simply don’t know whether any forecasters can predict something like the Our World in Data metrics 100 years from now with accuracy. The present may simply not provide enough intelligible information to predict the distant future with any confidence. There are a handful of historical figures from 100 years ago who seem to have been prescient about the world today, but it’s hard to know whether they were good forecasters or just lucky guessers. I suspect the same probabilistic judgment that allows good forecasters to make predictions about imminent and predictable futures may help them make more speculative forecasts. But forecasting the speculative future also belongs to the realm of science fiction because—to the extent that it’s even possible—it likely requires a broad imagination and an openness to possibilities that are unthinkable today.
Thanks to for recommending Telling the Future. In The Atlantic, Uri Friedman noted that Good Judgment superforecasters currently foresee "a long slog" ahead in Ukraine. I have a personal matter to attend to over the next couple of weeks, but I should be back at the end of the month with posts on the possibility of conflict between China and Taiwan and on the development of artificial intelligence. Telling the Future depends on the support of readers, so I’d appreciate it if you’d share it with others!
I am interested in your take on how much you think about the critique of forecasting itself being harmful in places with deep uncertainty.
I had read a lot of work previously on forecasting and incorporating "knightian uncertainty". However, I never truly understood how this breaks the notion of forecasting until I started getting uncomfortable with certain public forecasts.
Essentially, you probably don't even know what you want to forecast or what will drive the forecast so probabilities could lock in a sub par world view or sub par goal.
However! Good forecasters are strong at breaking apart a problem to the key drivers (at a point in time) and setting points where they would like to "reset" their forecast or change a policy/strategy.
I mean read the steps of a dynamic adaptive pathway
Step 1: Participatory problem framing, describe the system, specify objectives,and identify uncertainties
Step 2: Assess system vulnerabilities and opportunities, and identify adaptation tipping points
Step 3: Identify contingent actions and assess their ATP conditions and timing
Step 4: Design and evaluate pathways
Step 5: Design the adaptive strategy
That sounds a lot like how you build a strong forecast + some steps for actions as a result of the forecast.
I bring this up because I just appreciate this new world view for myself and I feel your piece above just suggests that forecasting under deep uncertainty requires a wide range of possibilities. Rather than a focus on the dynamic plan to deal with the wide range of possibilities.
I noticed you didn't mention any types of strategies for dealing with forecasting in deep uncertainty. Have you read "Decision Making under Deep Uncertainty". It seems related to the post and a de Neufville contributes a chapter.
https://library.oapen.org/handle/20.500.12657/22900