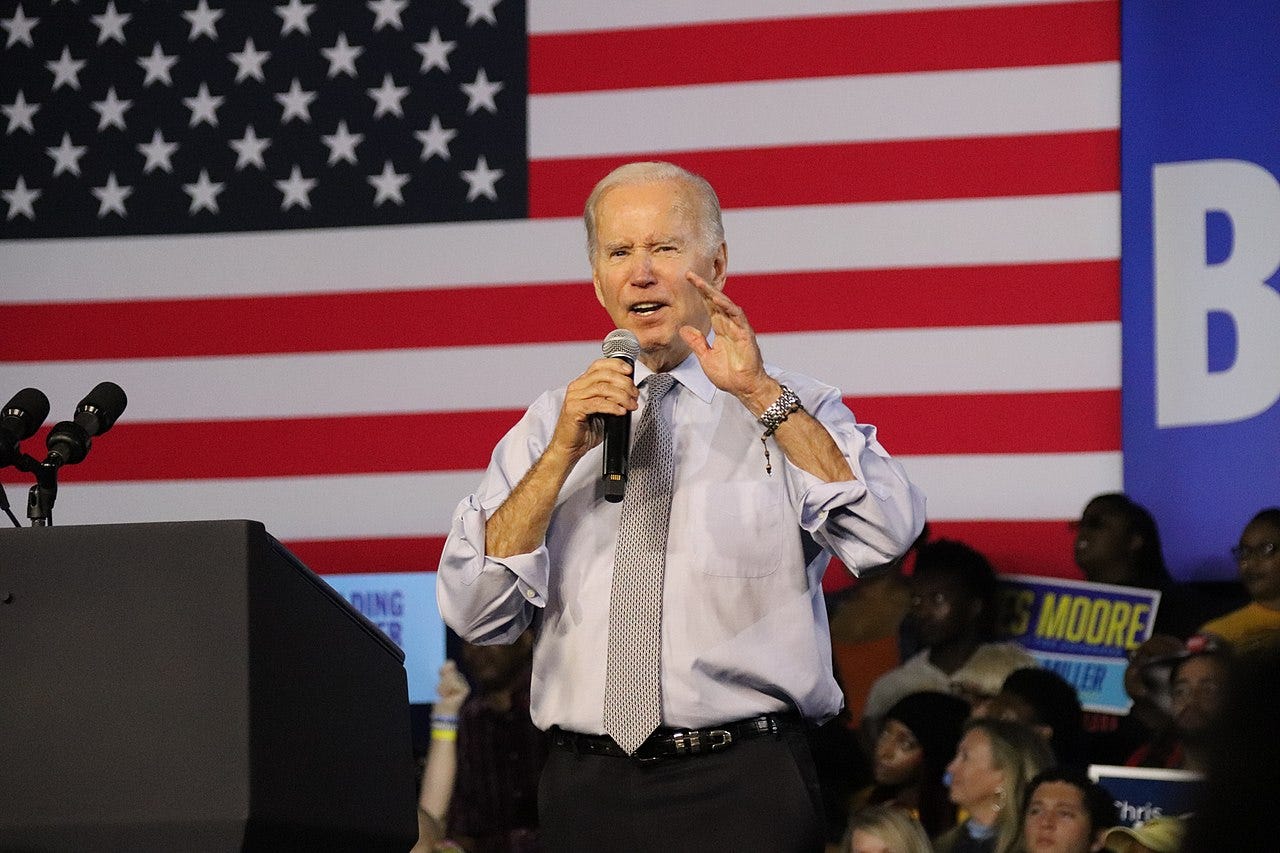
Simple rules—like that the party of power tends to lose seats in House in midterm elections—are surprisingly hard for forecasters to beat. In many cases, even subject matter experts don’t have enough information to reliably identify more subtle patterns. The hallmark of a good forecaster is not depth of knowledge, but the ability to judge when an inference is supported by the evidence.
You don’t need to know everything about a subject to make relatively accurate predictions about it. Forecasting requires a basic understanding of the field you’re trying to forecast—the kind of understanding a smart person can acquire through a few hours or days of diligent research—but you don’t need a graduate degree. In fact, subject matter experts, as a group, show no particular skill at forecasting within their own area of expertise.1 Whatever advantage their knowledge of a subject gives them is surprisingly small. The best forecasters are not those with the deepest knowledge, but those who are the most skilled at applying probability.
The truth is—as I’ve written before—it can be hard for forecasters to do much better than follow simple, reliable heuristics. Consider the rule that the party that controls the presidency during a midterm election almost always—18 of the last 19 times now—loses seats in the House of Representatives. Most people who follow American politics are aware of this historical pattern. If that was all you knew, you could have predicted that Republicans would probably gain seats in the House in November. You didn’t need to follow the polls, or know that inflation was high, or look at special election results. Having additional information might have helped—if you knew how to properly weigh it—but you didn’t need to build a deluxe forecasting model to know that Democrats were likely to lose seats in the House.

As Daniel Kahneman, Olivier Sibony, and Cass Sunstein explain their book Noise, simple heuristics can be hard to beat because we often don’t have enough data to improve on them.2 Robyn Dawes and Bernard Corrigan showed that for many predictive tasks, models that arbitrarily weight different factors equally are about as accurate as models that use regression analysis to determine which factors matter more.3 When there are a relatively small number of comparable events to draw on—there have been just 59 midterm elections in US history, for example—and a large number of potentially important factors, we can’t be confident any pattern we observe will necessarily hold in the future. In other words, when the available data is underpowered—where it doesn’t contain enough information to support a particular inference—arbitrary weights are about as likely to be about as accurate as complex models. As Dawes said, “we do not need models more precise than our measurements.”4
Subject matter experts may hold less of a forecasting advantage than we might expect because the additional knowledge they have adds relatively little predictive power to what amateurs already know. Experts may think their advanced knowledge gives them greater insight—or at least a different kind of insight—than it actually does. But the obscure connections experts are able draw are as likely to be statistical noise as they are to represent real causal relationships. Patterns that are robust enough to be statistically significant are likely to be intelligible to a diligent amateur.

We can sometimes improve on simple predictive rules if we're aware of the limitations of our knowledge. In some cases, it may be clear that a model isn’t accounting for some obvious factor. These cases are known as “broken-leg exceptions” after the idea that we should discount a reliable model of whether a person will go out to see movie that didn’t account for the fact that they just broke their leg.5 A human forecaster—or AI that has been trained on large amount of data—can identify these kind of unusual exceptions to otherwise reliable general rules. But many exceptions to the rule aren’t so obvious. Did the Supreme Court’s decision last year that there is no constitutional right to obtain an abortion constitute a broken-leg exception to the rule that the party out of power gains seats in the House in a midterm election? Recognizing real exceptions and identifying more subtle patterns is the hard challenge of forecasting. That's why the hallmark of a good forecaster is not depth of knowledge, but the ability to judge when an inference is supported by the evidence.
Congratulations to Metaculus on hosting one million forecasts. You can read about the Metaculus’ project for Our World in Data—which I worked on as a Metaculus Pro Forecaster—forecasting key measures of progress over the next 100 years here. Congratulations also to Superforecasting author and friend of Telling the Future , who has a new book with megaproject expert Bent Flyvbjerg on what makes projects succeed or fail called How Big Things Get Done. As always, if you’re enjoying my work you can support it by sharing it with others.

Great article Robert
One thing I’ve always liked about the forecasting community is that how it continuously calibrates itself against the goal of accuracy, which it also measures explicitly and clearly.
In other fields, you often see a trend of credentialised experts becoming obscurantist and vague when probed on the limits of their expertise. Perhaps they won’t even have a clear sense themselves of what the limits of their knowledge are. It’s rare to see the kind of meta norms that exist in forecasting, where experts are often characterised by a shrewd selfawareness and acknowledgement of what they can and can’t bring to the table.
I hope you are now doing well after your recent health issue.
The main weakness of simple rules is that in forecasting *competitions* you have to beat the average and not merely do well. This often means "cheating" and giving say a 93% event a 99% chance of happening, but it also sometimes means using more advanced techniques, but tbh I think the most underestimated technique is time-decay, where you say "will x happen by date Y" and you continuously move that probability downward as the date draws closer.
Near as I can tell there are roughly 10 or so basic forecasting techniques which when stated sound stupid but end up destroying the competition.
Namely
1. Read wikipedia for the base rate
2. Time decay your forecast
3. Incredible stuff rarely happens
4. The main way dictators get replaced is dying of old age
and I don't remember the rest off the top of my head